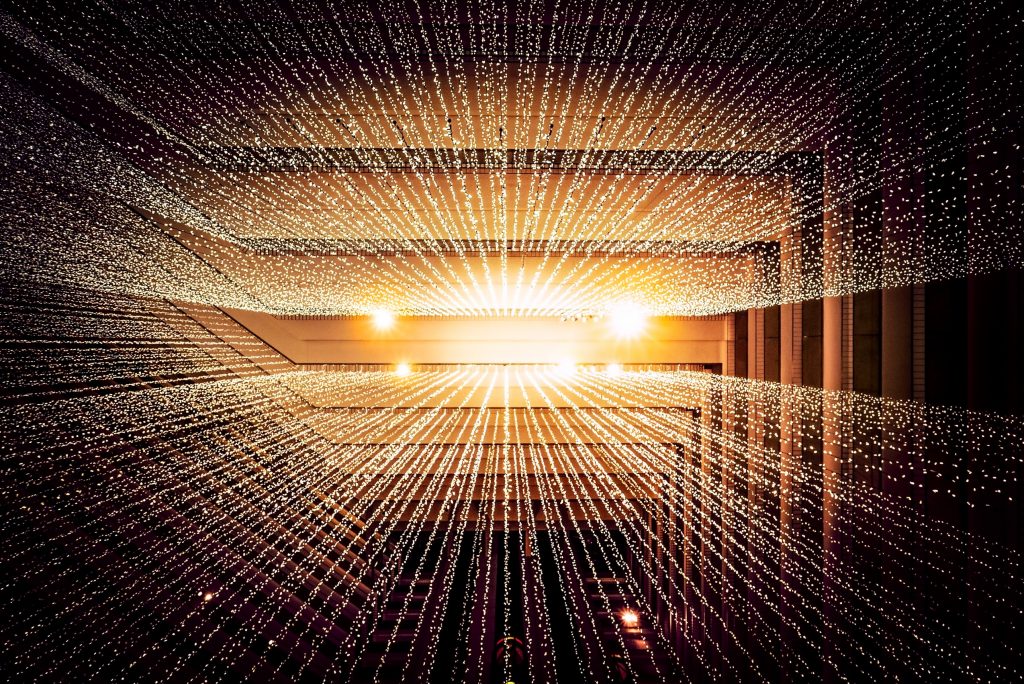
The Important Role of Data Mining in Supply Chain Operations
When businesses begin to scale, many of the challenges they face result from added operational complexity and lack of visibility. As organizations start investing more in their business’s functional components, such as inventory production, warehousing, logistics, and order management processing, communication silos and departmental disconnects begin to appear, blurring certain efficiency lines across the company as a whole.
To combat these operational challenges, many companies often rely on Key Performance Indicators (KPIs) to help them make informed decisions about their business as a whole. However, operational data in itself isn’t always useful in its raw state. Organizations typically need to take additional steps to make their system data informative and actionable. This is where data mining comes in.
What is Data Mining?
According to Consumer Notice, data mining is the process of turning raw data points into useful and actionable information. By collecting, sorting, and analyzing large amounts of data from various sources all at once, data mining helps companies discover valuable patterns and trends in their business operations.
Discovering and refining these data points manually across multiple systems would be time-consuming and inefficient for most businesses. Data mining simplifies the process exponentially and provides organizations with the unified data transparency they need to reduce their costs, improve client relationships, reduce operational risks, and increase revenues.
How Does Data Mining Work?
Much like the refining processes of metals and materials, data mining involves several stages before a final product can be achieved. When applying data mining in business analytics, the method uses the following six stages of progression:
- Business Understanding — Identification of business goals and recognize the overall outcome of the data mining process the organization wants to achieve.
- Data Discovery — The implementation of tools and solutions designed to understand all raw data formats and sources for the business and their relevance in the data mining process.
- Preparation — A key part of the “refining” process, often using AI-driven tools to convert unstructured raw data formats into forms that people can understand and quantify.
- Modeling — Several techniques are used to quickly and efficiently sort through several large databases simultaneously, identifying relevant trends and correlation analysis patterns.
- Evaluation — A series of human and machine-driven quality control processes to ensure data is properly mapped and accurately compiled.
- Deployment — Raw data has been fully refined and is now formatted to be used in near-limitless applications, whether represented as metrics, reports, or other digestible and actionable forms.
Data mining plays a fundamental role in business intelligence platforms and will continue to drive the future of data analytics as a whole. As businesses rely more and more on fast, actionable data to inform decisions around their growth and sustainability, data mining solutions will continue to be more readily adopted by all industries. In fact, data mining technology itself has already created roles within organizations such as data analytics specialists and data scientists who dedicate their professions to extracting and presenting new information to the business.
Data Mining in Supply Chain Operations
Management of a supply chain can be a daunting task for organizations of any size. Whether it’s running production facilities, coordinating shipping and logistics, managing inventory across multiple warehouses, or processing and tracking large volumes of orders, supply chains are made up of many individual components, each of them needing their own calibration efforts.
One area of supply chain management that heavily impacts business operations is product transportation. This is especially the case in the current landscape of remote business operations, now heavily reliant on shipping services and efficient vehicle routing. However, while the need for streamlined and profitable logistics coordination efforts has never been higher than it is now, many companies still use outdated and disconnected processes to keep things running.
Legacy logistics planning and tracking processes are often made up of many manual processes and riddled with routing problems that need solving. Some of these problems include inefficient shipping planning that leads to missed deadlines, a lack of visibility between shipment origin and destination, and unknown volumetric capacity or maintenance statuses of vehicles. With so much of these logistics processes disconnected from other critical components of the business, streamlining this data collection with other key business metrics is essential to ensure long-term business sustainability.
The data mining process does this by helping organizations create a unified view of all areas of the business. This is achieved through actionable reports highlighting key performance indicators, giving them the insight they need to improve how they manage product sourcing, efficient execution of their transportation network, and streamline all supporting workflows in and outside of the office.
The use cases of data mining are nearly limitless and can be applied to all business areas. However, by mining for data in supply chain operations, organizations can achieve the visibility they need to balance business efficiency, compliance, and profitability all in one place. This leads to a much more scalable business growth path and ensures long-term sustainability down the road.
*This article is written by guest author Beau Peters. View more of Beau’s articles here.