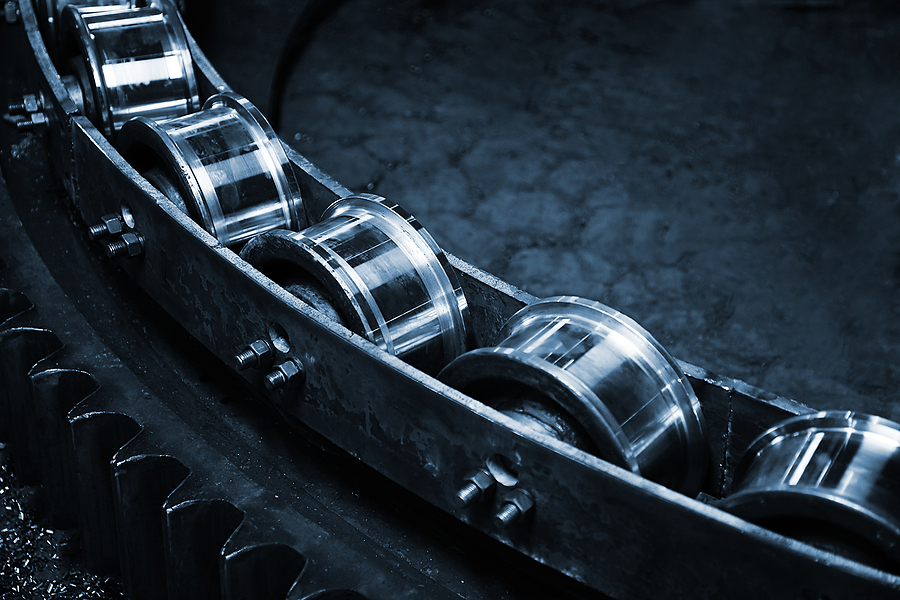
Variation Matters: Why AI Still Needs Humans for Accurate Predictive Analytics
Remember when artificial intelligence (AI) was a glimmer on the horizon? And then ChatGPT stormed onto the scene and people were convinced every job out there was soon going to be replaced by a bot? Now it turns out, not so much.
As awesome (and we don’t use that word lightly) as AI is, it’s only as good as the data it has to work with. At USC Consulting Group, we’re finding this is especially true when we’re using AI for predictive analytics. AI doesn’t like variation, and there can be a lot of that in manufacturing processes.
Here’s a look into this issue and how to handle it.
A short primer into AI and predictive analytics
AI is a broad term describing computer systems that perform intelligent tasks, like reasoning, learning, problem solving, and more. Not so obvious is predictive analytics, which is the ability to forecast future outcomes using AI based on data. You’re already familiar with it, to a certain degree. If you’ve ever had a recommendation from Netflix based on what you’ve watched in the past, that’s it. In a nutshell.
Netflix’s use of predictive analytics created a seismic shift in consumer expectations. This technology also has the potential to transform operating procedures and processes for many industries.
It’s extremely powerful when dealing with processes in which multiple predictors are influencing outcomes. It has the ability to tell us which path to take in order to achieve a desired outcome, even when process patterns and trends are changing.
It means greater precision and accuracy, speed and increased efficiency, the holy grails for any manufacturer.
But there is a fly in this cyber ointment.
Variation.
AI doesn’t like it and – low and behold – that means humans are necessary in this process in order for predictive analytics to achieve its potential.
What is variation?
When we’re talking about manufacturing processes, what exactly does variation mean?
In manufacturing, variation is the difference between an actual measure of a product characteristic and its target value. Excessive variation often leads to product discard or rework.
When you’re dealing with high process variation and instability, it degrades efficiency, consistency and ultimately, profits. A key manufacturing performance objective is the establishment of stable and predictable processes that limits variation – minimum variation around target values.
A main focus for USC Consulting Group is to identify the root causes of variation and address them. Generally, it boils down to people, components and materials.
Some examples to causes of variation include:
- Poor product design
- Poorly designed processes
- Unfit operations
- Unsuitable machines/equipment
- Untrained operators
- Variability from incoming vendor material
- Lack of adequate supervision skills
- Changing or inadequate environmental conditions
- Inadequate maintenance of equipment
- Inadequate or changing environmental conditions
It can be one of these factors, several, or something else. But whatever it is, it’s impeding our ability – and the bot’s – to predict outcomes.
Minimizing variation with our Customized Quality System (CQS)
Every situation is different. The cause of variation on one manufacturing line isn’t going to be the same on another. USCCG assesses and evaluates client processes, then applies a customized approach using a series of tools, techniques and methods that is most applicable in addressing the causes of variability. This customized approach enables USCCG to address variability in an efficient manner. We call it our Customized Quality System (CQS).
We review processes from “the cradle to the grave” and identify the highest-impact operations, then drill down to the tasks and steps within those operations until we uncover the culprits.
Although every situation is different, the general roadmap includes:
- Carefully defining the problem
- Selecting the right team
- Objectively identifying high-impact operations
- Drilling down into the tasks within those operations
- Brainstorming possible causes on those high-impact tasks
- Recommending and implementing deeply focused corrective actions
- Controls so it doesn’t happen again
Removing variability through our CQS not only has an immediate impact on improved product conformance but also paves the way for AI to do its job in predictive analytics, i.e., we want predictions with minimum variability.
It’s just one way USC Consulting Group is using the human touch to make sure AI is up to the job.
Read more about this in our free eBook, “AI and Machine Learning: Predicting the Future Through Analytics.”