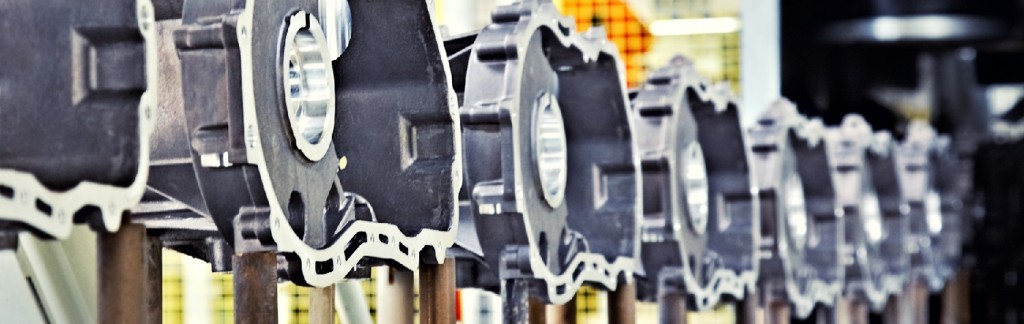
Enabling Process Improvements with ‘Objective-Based’ Data Management Tools
Innovation is driven by technological advancements, but far too often, manufacturers across all industries are overly concerned with how to bring the cutting edge to their products as opposed to their processes. The Big Data movement, canonized the world over as the saving grace for the burgeoning deluge of computerized information, allows businesses to do astronomically more in regards to customer relations and demand forecasting than once thought possible – that is, these companies can create a friction-less environment in which they can consume data at nigh-negligible costs, and apply their findings with minimal oversight and spend, resulting in momentous value-add potential.
But without the proper IT architecture in place, this can only be a pipe dream for businesses unwilling to bump up internal technological resources directly connected to production, be it the process or the product. Applications designed to monitor and control lean manufacturing initiatives can enhance the industries that bring them aboard, so long as these applications in question put the horse back before the cart, so to speak. What process improvements can be made to data-intensive operations using objective-minded management?
In manufacturing, measurement is everywhere, including data and process improvement.
Performance measurement
Believe it or not, metrics utilization isn’t just for the world of business – these days, the average individual uses a number of different “signals” to interact with the world around them, like how smartphone apps let hurried commuters know exactly what time public transportation pulls out of the station. In a sense, even low-tech devices like a car’s fuel gauges serve roughly the same purpose: conglomerating disparate information under a single, user-friendly visualization. In recent years, gas tank telemetry has evolved beyond simply stating fractions, but estimating how many miles a driver has left before refueling becomes a necessity. This is a much more actionable unit of measurement for this particular metric, as it assumes drivers won’t want to fill up unnecessarily as a means of saving money and time if it can be avoided without hindering functionality.
In a manufacturing plant, similar rules apply, but as industries grow more and more complex, production metrics continue to include more dimensions and incorporate larger volumes of data. However, the end results must be just as easy to understand and act on, perhaps even more so. Additionally, all manufacturers charting key performance indicators (KPIs) do so as these metrics relate to their own operations. Lean or Six Sigma only works so far as companies can identify their own shortcomings and apply these systems like antiseptic to a wound. According to a study by the faculty of manufacturing engineering at the Technical University of Malaysia, more often than not, KPIs “reflect the company’s mission, vision, objectives, and goal which are key imperatives to the company’s success,” especially when it comes to employee management. Any worthwhile application managing KPIs and alerting decision-makers as to vital data must therefore be as reliable as it is flexible to specific needs.
“Data from the end of a given process should inform operations at the very beginning.”
Process Improvements
Collecting and reporting on terabytes of data is one thing, but what can the right data management platform do to streamline tangible on-site workflow? Operational process improvements and efficiency can be gained through data management applications driven by lean manufacturing principles like kanban, or a system of inventory control where production information acts as a catalyst. The popular “pull, don’t push” paradigm used throughout manufacturing finds a home here as well. Data from the end of a given process should inform operations at the very beginning to promote efficient resource spend and temper production based on throughput potential. If a popular nightclub reaches capacity but the bouncer continues to allow guests entry, it creates an unsustainable and unsafe situation for everyone involved. However, if that same bouncer grants access based on how many guests leave, the venue can serve the most patrons without causing a commotion.
That said, as the Massachusetts Institute of Technology outlines, small discrepancies in different kanban-focused data management tools could actually stifle objective-based process improvements and detract from lean manufacturing practices. Certain lean manufacturing data management assets compartmentalize different actions in a given process and assign products within production as “carriers of information.” Returning to the nightclub analogy, if the bouncer has to occasionally go into the club and manually count occupants, this can create inefficiency and can convince people waiting in line for admission to seek out another place to spend their Friday night. But if that bouncer uses a counting device at the door, he or she can immediately respond to a happy party leaving as the impetus for allowing more people in. Similarly, manufacturers shouldn’t be “counting heads” so much as they should ensure their process improvements made to process management applications assign throughput data its proper role as a production instigator. In doing so, manufacturers avoid bottle-necked workflow and any resulting downtime, upgrading their efficiency through more intelligent datalogical deployments.